Distributed Autonomous Systems Laboratory
Prof. Girish Chowdhary
Prof. Girish Chowdhary is the director of Distribulted Autonomous Systems Laboratory(DASLAB) and Associate Professor and Donald Biggar Willet Faculty Fellow at the University of Illinois at Urbana-Champaign. He is the director of the USDA/NIFA Farm of the Future (I-FARM) site , a Co-PI on the AIFARMS National AI institute, and has a joint appointment between Department of Computer Science and Agricultural and Biological Engineering. He is a member of Coordinated Science Lab and is affiliated with Aerospace Engineering and Electrical and Computer Engineering.
Prof. Chowdhary was an Assistant Professor at the Department of Mechanical and Aerospace Engineering, Oklahoma State University for three years prior to moving to UIUC. He was a Postdoctoral Researcher at the Laboratory for Information and Decision Systems (LIDS) of the Massachusetts Institute of Technology.
Prof. Chowdhary has a Ph.D. degree from Georgia Institute of Technology. Prior to coming to Georgia Tech, he spent three years working as a research engineer with the German Aerospace Center's (DLR) Institute for Flight Systems Technology in Braunschweig, Germany. He holds a BE with honors from RMIT university in Melbourne, Australia.
Prof. Chowdhary is the author of several peer reviewed publications spanning the area of adaptive control, fault tolerant control, autonomy and decision making, machine learning, vision and LIDAR based perception for Unmanned Aerial Systems (UAS), and GPS denied navigation. He has been involved in the development of over 15 research unmanned aerial platforms.
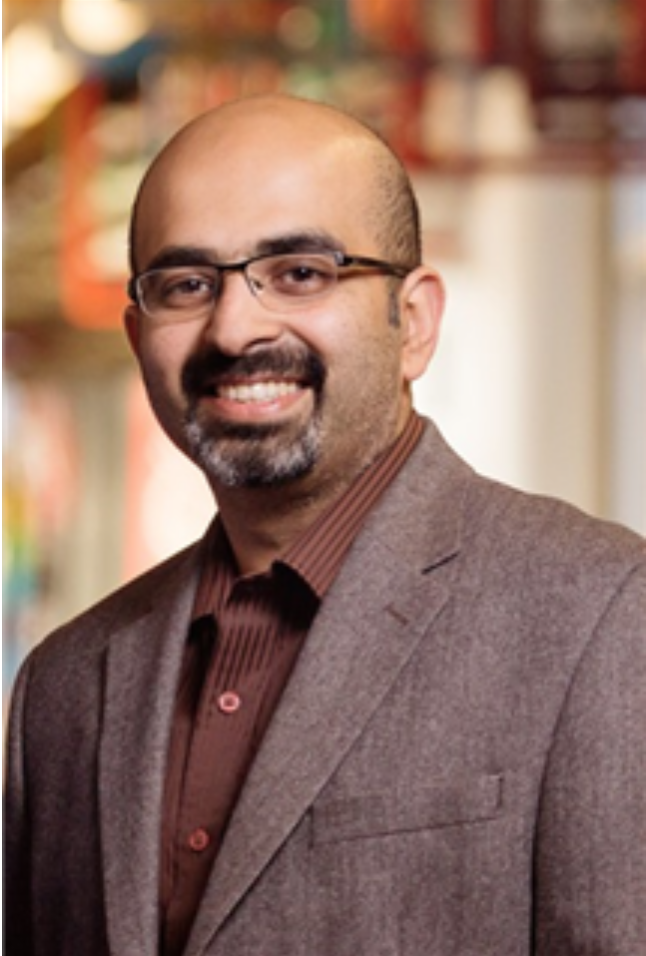
Meet Our Team
Naveen Kumar Uppalapati, Ph.D.
UIUC, USA
Anwesa Choudhuri
M. Ugur Akcal
Mateus Valverde Gasparino
Jiarui Sun
Faiza Aziz
Junzhe Wu
Kulbir Singh Ahluwalia
Shreya Guna Gummadi
José R. C. Valenzuela
Kendall Koe
Shivani Kamtikar
Scott Bout
Dennis McCann
Shaik Althaf
Pranav Yandamuri
Jiaming Xu
James Shin
Jimmy Fang
Joe Ku
Thomas Woehrle
Geonwoo Kim
Hassan Kingravi, Ph.D.
Pindrop Security, USA
Hossein Mohomadipanah, Ph.D.
University of Wisconsin, USA
Maximilian Muehlegg, Ph.D.
Audi GMbH, Germany
Erkan Kayacan, Ph.D.
Assistant Professor, The University of Oklahoma, Norman, OK
Zhongzhong Zhang, Ph.D.
Amazon, USA
Harshal Maske, Ph.D.
Ford Motor Company, USA
Denis Osipychev, Ph.D.
Boeing, USA
Girish Joshi, Ph.D.
MathWorks, USA
Wyatt McAllsiter, Ph.D.
HRI, Inc.
Sri Theja Vuppala, M.S.
EarthSense Inc., USA
Karan Chawla, M.S.
Elroy Air, USA
Sathwik M. Tejaswi, M.S.
Schneider National, USA
Hunter Young, M.S.
Intelligent Automation Inc., USA
Beau Barber, M.S.
UIUC, USA
Jasvir Virdi, M.S.
Nexteer Automotive, USA
Aaron Havens, M.S.
UIUC, USA
Garrett Thomas Gowan, M.S.
Aurora Innovation Inc., USA
Bhavana Jain, M.S.
Benjamin Thompson, B.S.
HCM Systems, USA
Volga Can Karakus, B.S.
EarthSense Inc., USA
Genevieve(Genny) Korn, B.S.
Micron Technology, USA
Billy Doherty, B.S.
EarthSense Inc., USA
Zhenwei(Selina) Wu, B.S.
Sahil Modi, B.S.
Joshua Varghese, B.S.
Aseem Vivek Borkar, Ph.D.
Engineering Manager at TIH-IoT, IIT Bombay
Nolan Replogle, M.S.
EarthSense Inc., USA
Yixiao Liu
Maximilian Oey
Corey Davidson, M.S.
The Boeing Company, USA
Andres Eduardo Baquero Velasquez, Ph.D.
EarthSense Inc., USA
Prabhat Kumar Mishra, Ph.D.
MIT, USA
Joshua Whitman
Teaching Fellowship, UIUC
Recent Publications
WayFASTER: a Self-Supervised Traversability Prediction for Increased Navigation Awareness
Mateus V Gasparino, Arun Narenthiran Sivakumar, Girish Chowdhary ICRA, 2024
Accurate and robust navigation in unstructured environments requires fusing data from multiple sensors. Such fusion ensures that the robot is better aware of its surroundings, including areas of the environment that are not immediately visible but were visible at a different time. To solve this problem, we propose a method for traversability prediction in challenging outdoor environments using a sequence of RGB and depth images fused with pose estimations. Our method, termed WayFASTER (Waypoints-Free Autonomous System for Traversability with Enhanced Robustness), uses experience data recorded from a receding horizon estimator to train a self-supervised neural network for traversability prediction, eliminating the need for heuristics. Our experiments demonstrate that our method excels at avoiding obstacles, and correctly detects that traversable terrains, such as tall grass, can be navigable. By using a sequence of images, WayFASTER significantly enhances the robot's awareness of its surroundings, enabling it to predict the traversability of terrains that are not immediately visible. This enhanced awareness contributes to better navigation performance in environments where such predictive capabilities are essential.
Unmatched Uncertainty Mitigation Through Neural Network Supported Model Predictive Control
Mateus V Gasparino, Prabhat K Mishra, Girish Chowdhary CDC, 2023
This paper presents a deep learning based model predictive control (MPC) algorithm for systems with unmatched and bounded state-action dependent uncertainties of unknown structure. We utilize a deep neural network (DNN) as an oracle in the underlying optimization problem of learning based MPC (LBMPC) to estimate unmatched uncertainties. Generally, non-parametric oracles such as DNN are considered difficult to employ with LBMPC due to the technical difficulties associated with estimation of their coefficients in real time. We employ a dual-timescale adaptation mechanism, where the weights of the last layer of the neural network are updated in real time while the inner layers are trained on a slower timescale using the training data collected online and selectively stored in a buffer. Our results are validated through a numerical experiment on the compression system model of jet engine. These results indicate that the proposed approach is implementable in real time and carries the theoretical guarantees of LBMPC.
NDVI/NDRE Prediction from Standard RGB Aerial Imagery Using Deep Learning
Corey Davidson, Vishnu Jaganathan, Arun Narenthiran Sivakumar, Joby M. Prince Czarnecki, Girish Chowdhary Computers and Electronics in Agriculture, 2023
The growth of precision agriculture has allowed farmers access to more data and greater efficiency for their farms. With consistently tight profit margins, farmers need ways to take advantage of the advancement of technology to lower their costs or increase their revenue. One area where these advancements can prove beneficial are in the measurement of vegetation indices such as the Normalized Difference Vegetation Index (NDVI) and Normalized Difference Red Edge Index (NDRE). Color maps representing these vegetation indices can be used to identify problem areas, plant health, or even places where spot applications are needed. These color maps help farmers to visualize these areas. Currently, a multi-thousand dollar multispectral camera, typically attached to an Unmanned Aerial Vehicle (UAV) during flight, is required for measuring these indices. This makes obtaining NDVI and NDRE somewhat cost prohibitive for most farmers. This work demonstrates a solution to this cost issue. The solution involves the use of a conditional Generative Adversarial Network known as Pix2Pix. By using Pix2Pix along with training data from UAV flights of corn, soybeans, and cotton, this paper highlights the potential for predicting comparable NDVI and NDRE with a low-cost Red-Green-Blue (RGB) camera. This paper proposes and assesses a cost-efficient method that can comparably predict these vegetation indices, resulting in cost-savings in the range of $5000 per UAV system.
Under-Canopy Dataset for Advancing Simultaneous Localization and Mapping in Agricultural Robotics
Jose Cuaran, Andres Eduardo Baquero Velasquez, Mateus Valverde Gasparino, Naveen Kumar Uppalapati, Arun Narenthiran Sivakumar, Justin Wasserman, Muhammad Huzaifa, Sarita Adve, Girish Chowdhary International Journal of Robotics Research, 2023
Simultaneous localization and mapping (SLAM) has been an active research problem over recent decades. Many leading solutions are available that can achieve remarkable performance in environments with familiar structure, such as indoors and cities. However, our work shows that these leading systems fail in an agricultural setting, particularly in under the canopy navigation in the largest-in-acreage crops of the world: corn (Zeamays) and soybean (Glycinemax). The presence of plenty of visual clutter due to leaves, varying illumination, and stark visual similarity makes these environments lose the familiar structure on which SLAM algorithms rely on. To advance SLAM in such unstructured agricultural environments, we present a comprehensive agricultural dataset. Our open dataset consists of stereo images, IMUs, wheel encoders, and GPS measurements continuously recorded from a mobile robot in corn and soybean fields across different growth stages. In addition, we present best-case benchmark results for several leading visual-inertial odometry and SLAM systems. Our data and benchmark clearly show that there is significant research promise in SLAM for agricultural settings.
Last-Mile Embodied Visual Navigation
Justin Wasserman*, Karmesh Yadav, Girish Chowdhary, Abhinav Gupta, Unnat Jain* CORL, 2022
Realistic long-horizon tasks like image-goal navigation involve exploratory and exploitative phases. Assigned with an image of the goal, an embodied agent must explore to discover the goal, i.e., search efficiently using learned priors. Once the goal is discovered, the agent must accurately calibrate the last-mile of navigation to the goal. As with any robust system, switches between exploratory goal discovery and exploitative last-mile navigation enable better recovery from errors. Following these intuitive guide rails, we propose SLING to improve performance of existing image-goal navigation systems. Entirely complementing prior methods, we focus on last-mile navigation, and leverage the underlying geometric structure of the problem with neural descriptors. With simple but effective switches, we can easily connect SLING with heuristic, reinforcement learning, and neural modular policies. On a standardized image-goal navigation benchmark, we improve performance across policies, scenes, and episode complexity, raising the state-of-the-art from 45% to 55% success rate and 28.0% to 37.4% SPL. Beyond photorealistic simulation, we conduct real-robot experiments in three physical scenes and find these improvements to transfer well to real environments.
WayFAST: Navigation with Predictive Traversability in the Field
Mateus Valverde Gasparino, Arun Narenthiran Sivakumar, Yixiao Liu, Andres Eduardo Baquero Velasquez, Vitor Akihiro Hisano Higuti, John Rogers, Huy Tran, Girish Chowdhary IROS, 2022
We present a self-supervised approach for learning to predict traversable paths for wheeled mobile robots that require good traction to navigate. Our algorithm, termed WayFAST (Waypoint Free Autonomous Systems for Traversability), uses RGB and depth data, along with navigation experience, to autonomously generate traversable paths in outdoor unstructured environments. Our key inspiration is that traction can be estimated for rolling robots using kinodynamic models. Using traction estimates provided by an online receding horizon estimator, we are able to train a traversability prediction neural network in a self-supervised manner, without requiring heuristics utilized by previous methods. We demonstrate the effectiveness of WayFAST through extensive field testing in varying environments, ranging from sandy dry beaches to forest canopies and snow covered grass fields. Our results clearly demonstrate that WayFAST can learn to avoid geometric obstacles as well as untraversable terrain, such as snow, which would be difficult to avoid with sensors that provide only geometric data, such as LiDAR. Furthermore, we show that our training pipeline based on online traction estimates is more data-efficient than other heuristic-based methods.
Visual Servoing for Pose Control of Soft Continuum Arm in a Structured Environment
Shivani Kiran Kamtikar, Samhita Marri, Benjamin Thomas Walt, Naveen Kumar Uppalapati, Girish Krishnan, Girish Chowdhary RoboSoft-RAL, 2022
For soft continuum arms, visual servoing is a popular control strategy that relies on visual feedback to close the control loop. However, robust visual servoing is challenging as it requires reliable feature extraction from the image, accurate control models and sensors to perceive the shape of the arm, both of which can be hard to implement in a soft robot. This letter circumvents these challenges by presenting a deep neural network-based method to perform smooth and robust 3D positioning tasks on a soft arm by visual servoing using a camera mounted at the distal end of the arm. A convolutional neural network is trained to predict the actuations required to achieve the desired pose in a structured environment. Integrated and modular approaches for estimating the actuations from the image are proposed and are experimentally compared. A proportional control law is implemented to reduce the error between the desired and current image as seen by the camera. The model together with the proportional feedback control makes the described approach robust to several variations such as new targets, lighting, loads, and diminution of the soft arm. Furthermore, the model lends itself to be transferred to a new environment with minimal effort.
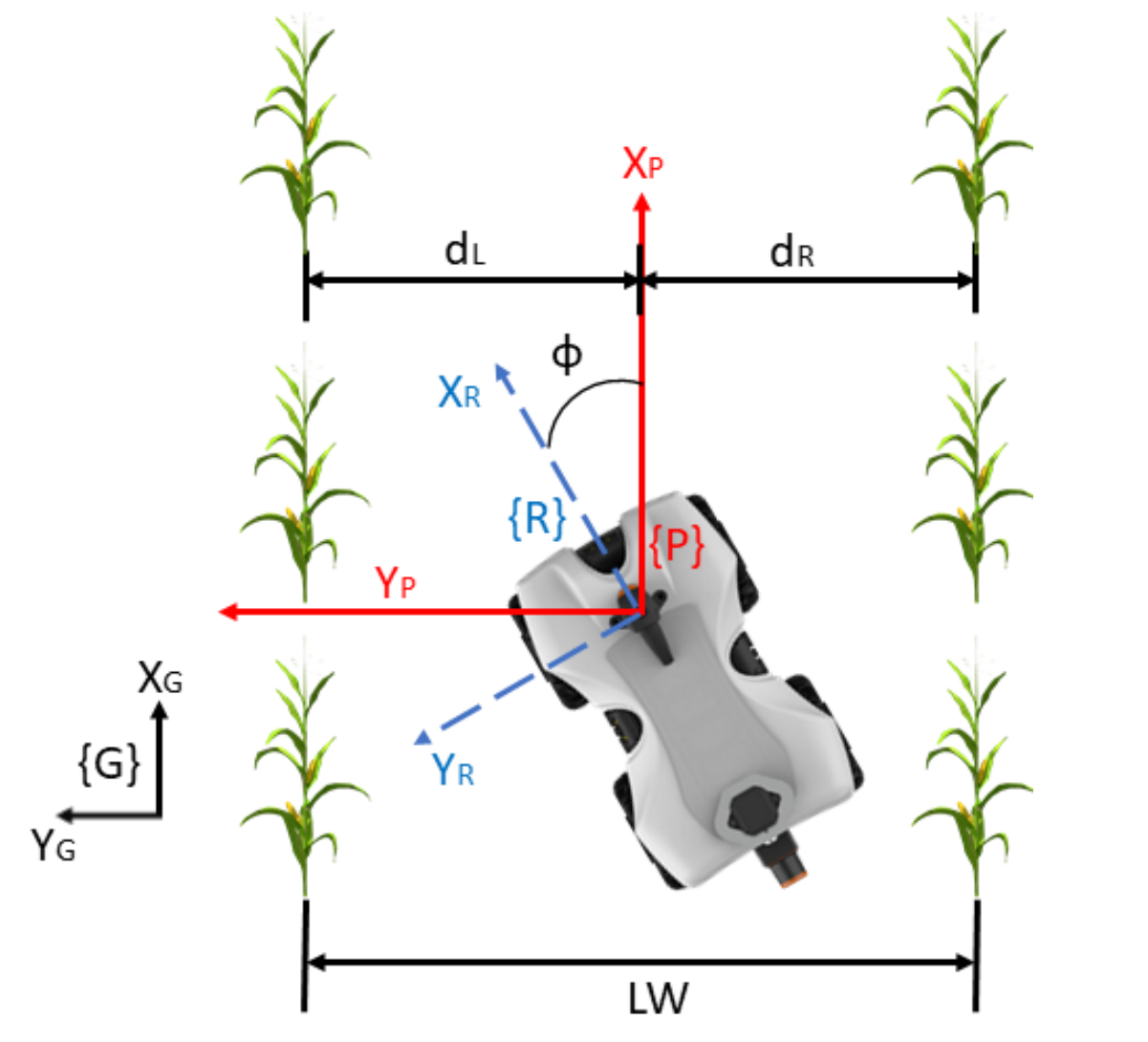
Multi-Sensor Fusion based Robust Row Following for Compact Agricultural Robots
Andres Eduardo Baquero Velasquez, Vitor Akihiro Hisano Higuti, Mateus Valverde Gasparino, Arun Narenthiran Sivakumar, Marcelo Becker, Girish Chowdhary Field Robotics, 2022
This paper presents a state-of-the-art LiDAR based autonomous navigation system for under-canopy agricultural robots. Under-canopy agricultural navigation has been a challenging problem because GNSS and other positioning sensors are prone to significant errors due to attentuation and multi-path caused by crop leaves and stems. Reactive navigation by detecting crop rows using LiDAR measurements is a better alternative to GPS but suffers from challenges due to occlusion from leaves under the canopy. Our system addresses this challenge by fusing IMU and LiDAR measurements using an Extended Kalman Filter framework on low-cost hardwware. In addition, a local goal generator is introduced to provide locally optimal reference trajectories to the onboard controller. Our system is validated extensively in real-world field environments over a distance of 50.88~km on multiple robots in different field conditions across different locations. We report state-of-the-art distance between intervention results, showing that our system is able to safely navigate without interventions for 386.9~m on average in fields without significant gaps in the crop rows, 56.1~m in production fields and 47.5~m in fields with gaps (space of 1~m without plants in both sides of the row).
Assignment-Space-based Multi-Object Tracking and Segmentation
Anwesa Choudhuri, Girish Chowdhary, Alexander G. Schwing International Conference on Computer Vision (ICCV), 2021
Multi-object tracking and segmentation (MOTS) is important for understanding dynamic scenes in video data. Existing methods perform well on multi-object detection and segmentation for independent video frames, but tracking of objects over time remains a challenge. MOTS methods formulate tracking locally, i.e., frame-by-frame, leading to sub-optimal results. Classical global methods on tracking operate directly on object detections, which can lead to a combinatorial growth in the detection space. To address these issues, we formulate a global method for MOTS over the space of assignments rather than detections. In step 1 of our two step method we find all top-k assignments of objects detected and segmented between any two consecutive frames. We then develop a structured prediction formulation to score assignment sequences across any number of consecutive frames and use dynamic programming to find the global optimizer of the structured prediction formulation in polynomial time. In step 2 we connect objects which reappear after having been out of view for some time. For this we formulate an assignment problem. On the challenging KITTI-MOTS and MOTSChallenge datasets, this method achieves state-of-the-art results among methods which don’t use depth information.
Learned Visual Navigation for Under-Canopy Agricultural Robots
Arun Sivakumar, Sahil Modi, Mateus Gasparino, Che Ellis, Andres Velasquez, Girish Chowdhary*, Saurabh Gupta* Robotics: Science and Systems (RSS), 2021
We describe a system for visually guided autonomous navigation of under-canopy farm robots. Low-cost under-canopy robots can drive between crop rows under the plant canopy and accomplish tasks that are infeasible for over-the-canopy drones or larger agricultural equipment. However, autonomously navigating them under the canopy presents a number of challenges: unreliable GPS and LiDAR, high cost of sensing, challenging farm terrain, clutter due to leaves and weeds, and large variability in appearance over the season and across crop types. We address these challenges by building a modular system that leverages machine learning for robust and generalizable perception from monocular RGB images from low-cost cameras, and model predictive control for accurate control in challenging terrain. Our system, CropFollow, is able to autonomously drive 485 meters per intervention on average, outperforming a state-of-the-art LiDAR based system (286 meters per intervention) in extensive field testing spanning over 25 km.
Agbots 3.0: Adaptive Weed Growth Prediction for Mechanical Weeding Agbots
Wyatt McAllister, Joshua Whitman, Joshua Varghese, Adam Davis, Girish Chowdhary IEEE Transactions on Robotics, 2021
This work presents advances in predictive modeling of weed growth, as well as an improved planning index to be used in conjunction with these techniques, for the purpose of improving the performance of coordinated weeding algorithms being developed for industrial agriculture. We demonstrate that the evolving Gaussian process (E-GP) method applied to measurements from the agents can predict the evolution of the field within the realistic simulation environment, Weed World. This method also provides physical insight into the seed bank distribution of the field. In this work, we extend the E-GP model in two important ways. First, we have developed a model that has a bias term, and we show how it is connected to the seed bank distribution. Second, we show that one may decouple the component of the model representing weed growth from the component, which varies with the seed bank distribution, and adapt the latter online. We compare this predictive approach with one that relies on known properties of the weed growth model and show that the E-GP method can drive down the total weed biomass for fields with high seed bank densities using less agents, without assuming this model information. We use an improved planning index, the Whittle index, which allows a balanced tradeoff between exploiting a row or allowing it to accrue reward and conforms to what we show is the theoretical limit for the fewest number of agents, which can be used in this domain.
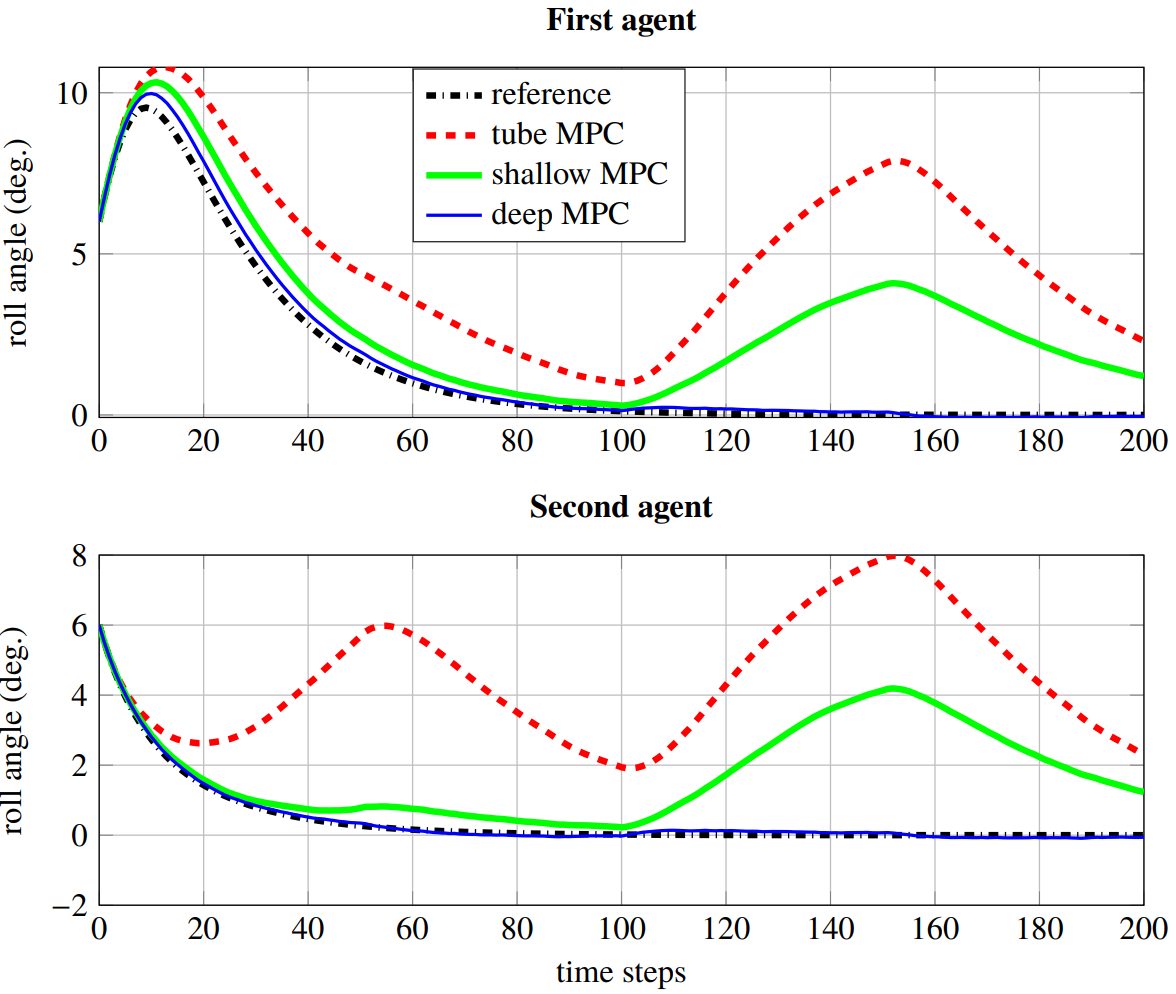
Deep Model Predictive Control for a Class of Nonlinear Systems
Prabhat K. Mishra, Mateus V. Gasparino, Andres E. B. Velsasquez, Girish Chowdhary Under Review
A control affine nonlinear discrete time system is considerd with mached and bounded state dependent uncertainties. Since the structure of uncertainties is not known, a deep learning based adaptive mechanism is utilized to encounter disturbances. In order to avoid any unwanted behavior during the learning phase, a tube based model predictive controller is employed, which ensures satisfaction of constraints and input-to-state stability of the closed-loop states. In addition, the proposed approach is applicable to multi-agent systems with non-interacting agents and a centralized controller.
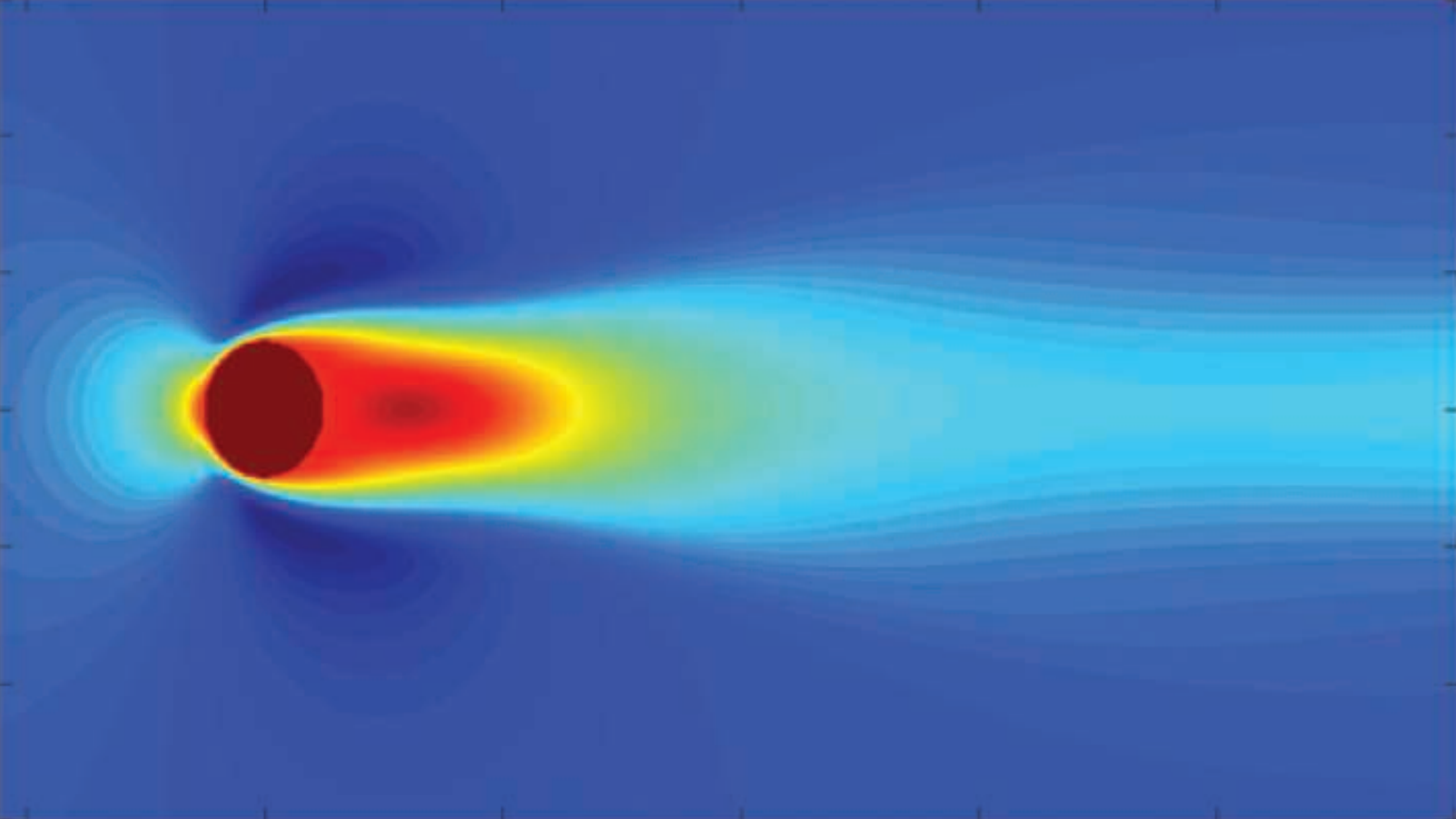
Joshua E. Whitman, Harshal Maske, Hassan A. Kingravi, Girish Chowdhary IEEE Control Systems Magazine, 2021
This article should be useful for anyone interested in using robots in large-scale environments that are changing in time and space. The methods presented here have been used to help teams of robots monitor and destroy weeds within a field of crops. In general, this article discusses modeling and monitoring complex systems that vary in both space and time, given a limited number of agents or sensors providing measurements spread out across a large area. A novel method for solving this problem is presented, with several tremendously useful properties. First, it can be easily trained and updated even with large, “dirty” data collected at many places at many times. Second, this model lends itself well to the kinds of analyses familiar to the controls community, which means that several formerly very challenging problems become much easier (for example, predicting future evolution, deciding how many sensors are needed and where to place them, and determining the basic structures beneath the system dynamics). As far as it is possible to tell, the methods presented here are unmatched in their scope and power. A graduate-level mathematical background is recommended for this article, and an open code repository is provided for the ease of implementation.
Multi-Modal Anomaly Detection for Unstructured and Uncertain Environments
Tianchen Ji, Sri Theja Vuppala, Girish Chowdhary, Katherine Driggs-Campbell Conference on Robot Learning, 2020
To achieve high-levels of autonomy, modern robots require the ability to detect and recover from anomalies and failures with minimal human supervision. Multi-modal sensor signals could provide more information for such anomaly detection tasks; however, the fusion of high-dimensional and heterogeneous sensor modalities remains a challenging problem. We propose a deep learning neural network: supervised variational autoencoder (SVAE), for failure identification in unstructured and uncertain environments. Our model leverages the representational power of VAE to extract robust features from high-dimensional inputs for supervised learning tasks. The training objective unifies the generative model and the discriminative model, thus making the learning a one-stage procedure. Our experiments on real field robot data demonstrate superior failure identification performance than baseline methods, and that our model learns interpretable representations.
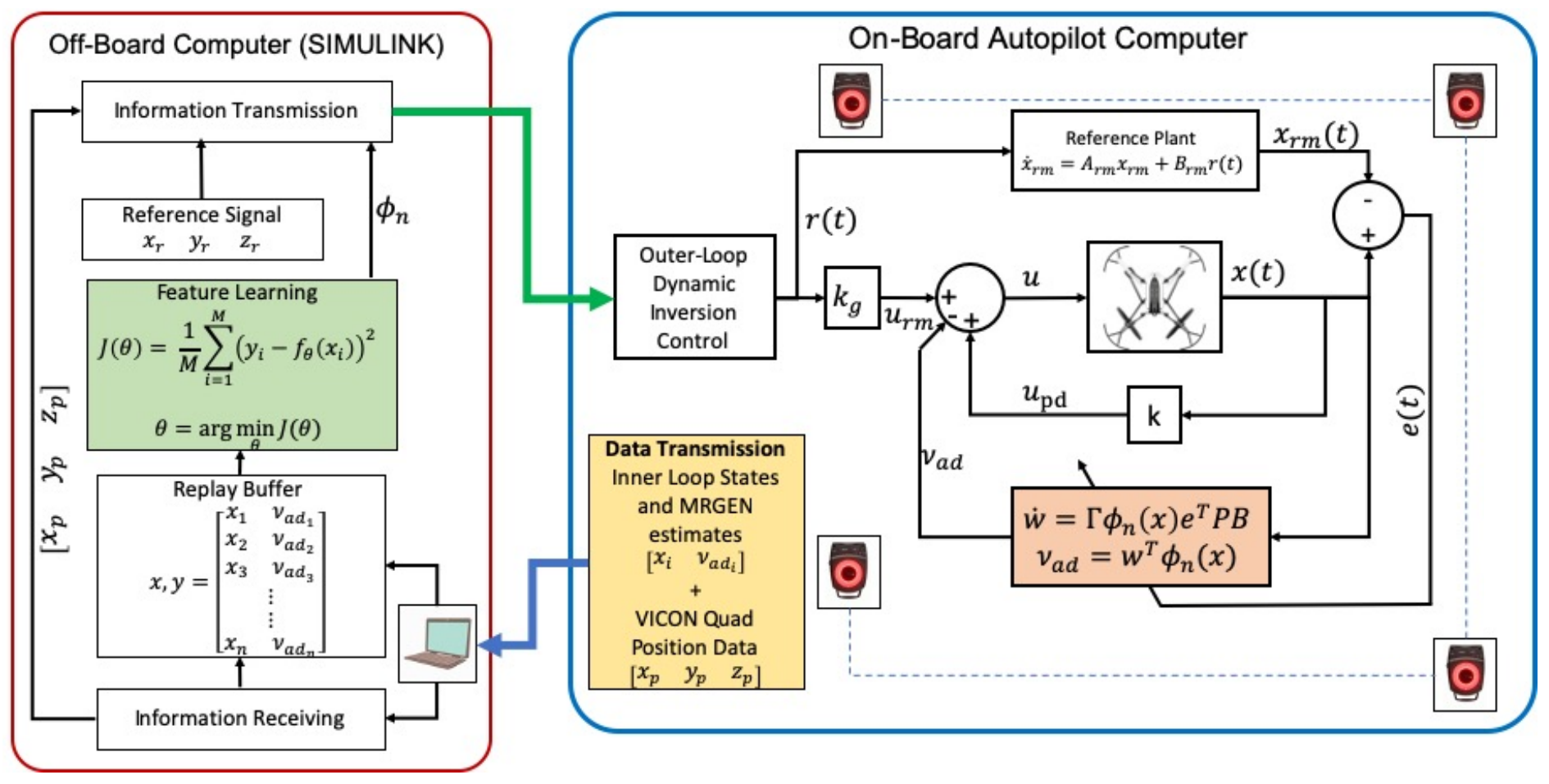
Asynchronous Deep Model Reference Adaptive Control
Girish Joshi, Jasvir Virdi, Girish Chowdhary Conference on Robot Learning, 2020
In this paper, we present Asynchronous implementation of Deep Neural Network-based Model Reference Adaptive Control (DMRAC). We evaluate this new neuro-adaptive control architecture through flight tests on a small quadcopter. We demonstrate that a single DMRAC controller can handle significant nonlinearities due to severe system faults and deliberate wind disturbances while executing high-bandwidth attitude control. We also show that the architecture has long-term learning abilities across different flight regimes, and can generalize to fly different flight trajectories than those on which it was trained. These results demonstrating the efficacy of this architecture for high bandwidth closed-loop attitude control of unstable and nonlinear robots operating in adverse situations. To achieve these results, we designed a software+communication architecture to ensure online real-time inference of the deep network on a high-bandwidth computation-limited platform. We expect that this architecture will benefit other deep learning in the closed-loop experiments on robots.
A Berry Picking Robot With A Hybrid Soft-Rigid Arm: Design and Task Space Control
Naveen Kumar Uppalapati, Benjamin Walt, Aaron Havens, Armeen Mahdian, Girish Chowdhary, Girish Krishnan Robotics: Science and Systems (RSS), 2020
We present a hybrid rigid-soft arm and manipulator for performing tasks requiring dexterity and reach in cluttered environments. Our system combines the benefit of the dexterity of a variable length soft manipulator and the rigid support capability of a hard arm. The hard arm positions the extendable soft manipulator close to the target, and the soft arm manipulator navigates the last few centimeters to reach and grab the target. A novel magnetic sensor and reinforcement learning based control is developed for end effector position control of the robot. A compliant gripper with an IR reflectance sensing system is designed, and a k-nearest neighbor classifier is used to detect target engagement. The system is evaluated in several challenging berry picking scenarios.
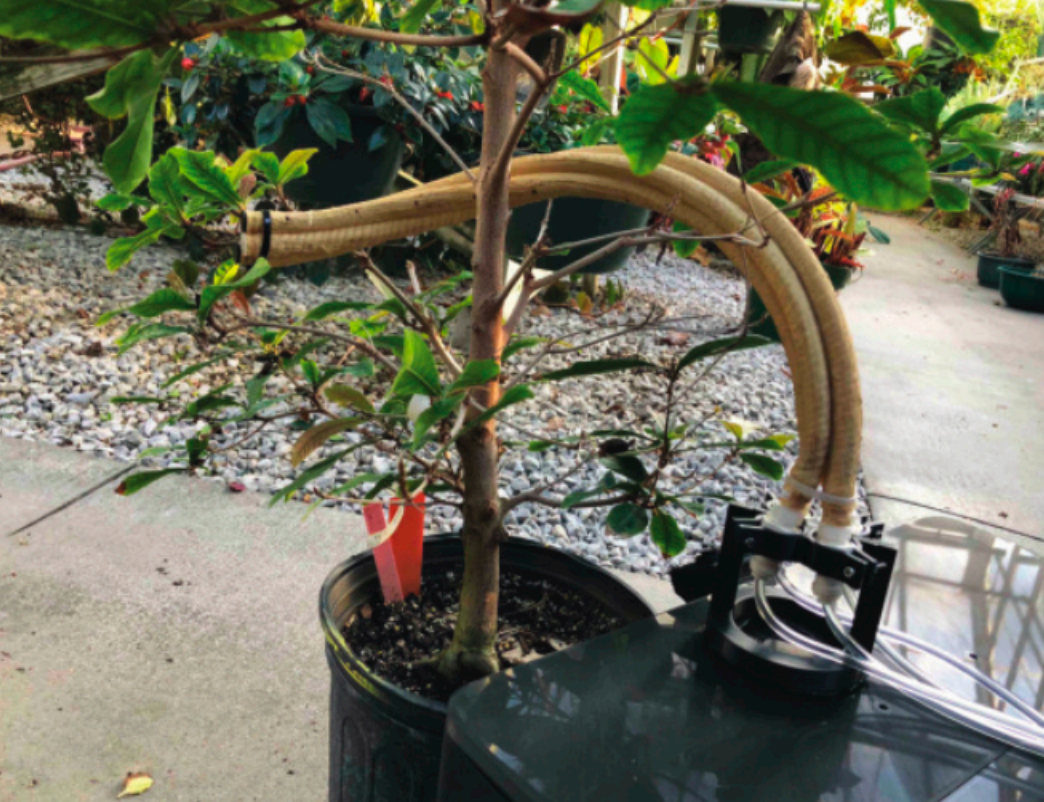
Soft Robotics as an Enabling Technology for Agroforestry Practice and Research
Girish Chowdhary, Mattia Gazzola, Girish Krishnan, Chinmay Soman, Sarah Lovell MDPI Sustainability, 2019
The shortage of qualified human labor is a key challenge facing farmers, limiting profit margins and preventing the adoption of sustainable and diversified agroecosystems, such as agroforestry. New technologies in robotics could offer a solution to such limitations. Advances in soft arms and manipulators can enable agricultural robots that can have better reach and dexterity around plants than traditional robots equipped with hard industrial robotic arms. Soft robotic arms and manipulators can be far less expensive to manufacture and significantly lighter than their hard counterparts. Furthermore, they can be simpler to design and manufacture since they rely on fluidic pressurization as the primary mechanisms of operation. However, current soft robotic arms are difficult to design and control, slow to actuate, and have limited payloads. In this paper, we discuss the benefits and challenges of soft robotics technology and what it could mean for sustainable agriculture and agroforestry.
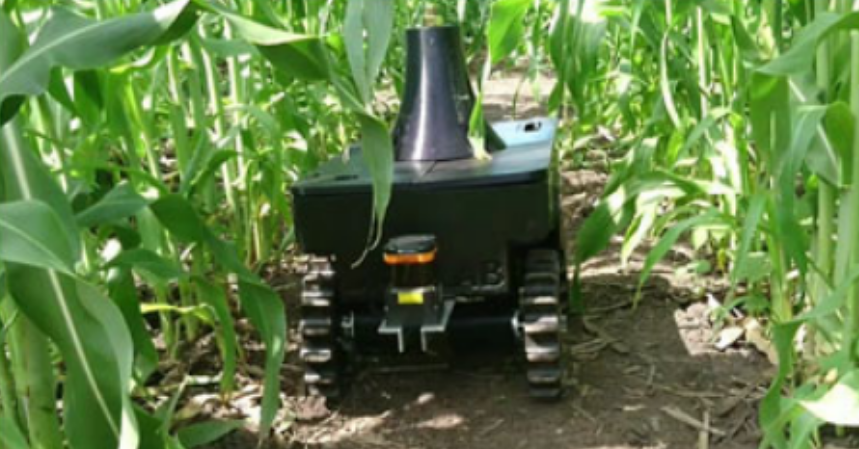
Under Canopy Light Detection and Ranging Based Autonomous Navigation
Vitor A. H. Higuti, Andres E. B. Velasquez, Daniel Varela Magalhaes, Marcelo Becker, Girish Chowdhary Journal of Field Robotics, 2018
This paper describes a light detection and ranging (LiDAR)-based autonomous navigation system for an ultralightweight ground robot in agricultural fields. The system is designed for reliable navigation under cluttered canopies using only a 2D Hokuyo UTM-30LX LiDAR sensor as the single source for perception. Its purpose is to ensure that the robot can navigate through rows of crops without damaging the plants in narrow row-based and high-leaf-cover semistructured crop plantations, such as corn (Zea mays) and sorghum ( Sorghum bicolor). The key contribution of our work is a LiDAR-based navigation algorithm capable of rejecting outlying measurements in the point cloud due to plants in adjacent rows, low-hanging leaf cover or weeds. The algorithm addresses this challenge using a set of heuristics that are designed to filter out outlying measurements in a computationally efficient manner, and linear least squares are applied to estimate within-row distance using the filtered data. Moreover, a crucial step is the estimate validation, which is achieved through a heuristic that grades and validates the fitted row-lines based on current and previous information. The proposed LiDAR-based perception subsystem has been extensively tested in production/breeding corn and sorghum fields. In such variety of highly cluttered real field environments, the robot logged more than 6 km of autonomous run in straight rows. These results demonstrate highly promising advances to LiDAR-based navigation in realistic field environments for small under-canopy robots.
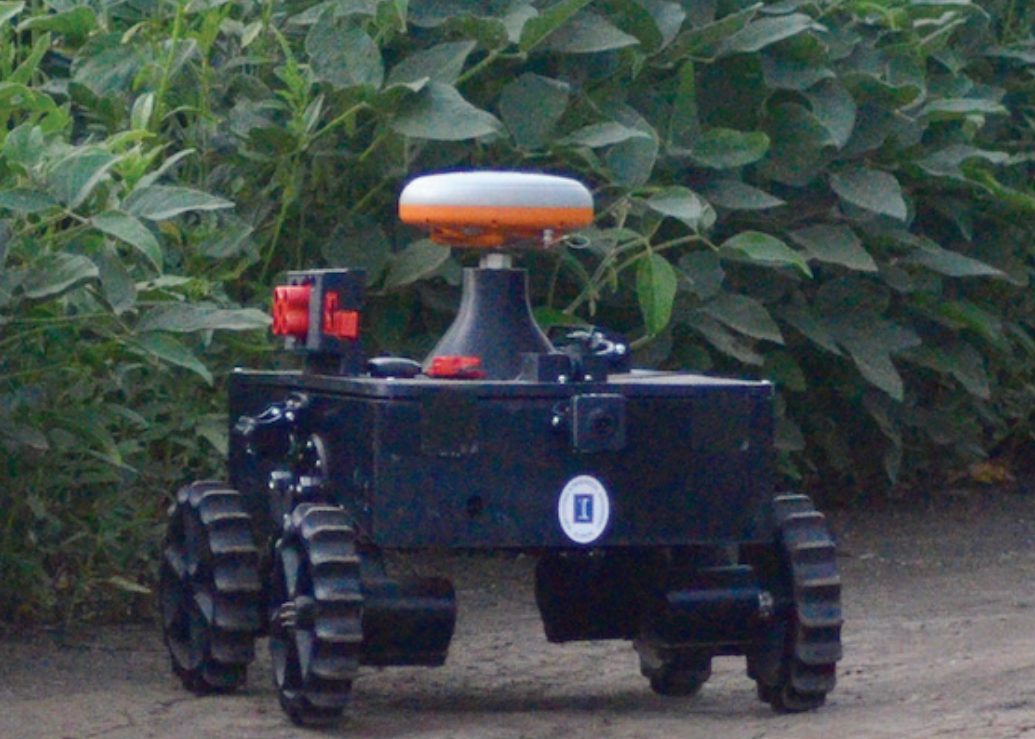
Erkan Kayacan, Zhongzhong Zhang, Girish Chowdhary Robotics: Science and Systems (RSS), 2018 (Best Paper Award)
This paper presents embedded high precision control and corn stands counting algorithms for a low-cost, ultra-compact 3D printed and autonomous field robot for agricultural operations. Currently, plant traits, such as emergence rate, biomass, vigor and stand counting are measured manually. This is highly labor intensive and prone to errors. The robot, termed TerraSentia, is designed to automate the measurement of plant traits for efficient phenotyping as an alternative to manual measurements. In this paper, we formulate a nonlinear moving horizon estimator that identifies key terrain parameters using onboard robot sensors and a learning-based nonlinear model predictive control (NMPC) that ensures high precision path tracking in the presence of unknown wheel-terrain interaction. Moreover, we develop a machine vision algorithm to enable TerraSentia to count corn stands by driving through the fields autonomously. We present results of an extensive field-test study that shows that i) the robot can track paths precisely with less than 5cm error so that the robot is less likely to damage plants, and ii) the machine vision algorithm is robust against interferences from leaves and weeds, and the system has been verified in corn fields at the growth stage of V4, V6, VT, R2, and R6 from five different locations. The robot predictions agree well with the ground truth with the correlation coefficient R=0.96.
VIDEOS
We've been in the NEWS!!!
Our Software
Get in touch
Say Hello
Email: Dr. Girish ChowdharyEmail: Dr. Naveen Kumar Uppalapati
Email: Samhita Marri (Web Master)